Since its inception, machine learning has had innumerable impacts on organizations, industries, and business ecosystems globally. Several high-magnitude examples of recombinant innovation are powered by machine learning applications in conjunction with big data, robotics, and the Internet of Things.
With a precise introduction out of the way, let’s explore the myriad integration of machine learning in organizations, the impact this technology has over various markets, and how the C-suite can deploy this technology in their industries.
What is Machine Learning?
Machine learning is a subset application of artificial intelligence in which computers self–learn and perform actions based on a given database without programming.
Machine Learning: The Industry Insights
- 71% of research and development professionals said that they have already deployed big data and machine learning in their business operations. This is the fastest department to deploy ML.
- Customer experience improvement represents 57% of the use cases of artificial intelligence and machine learning (Statista).
- AI and ML algorithms can improve the global GDP up to 14% by 2030, which is equivalent to approximately 14 trillion dollars (Wall Street Journal).
10 Applications of Machine Learning
Applications of machine learning have created transformative differences in every business field we can think of. From healthcare to space explorations, machine learning has boosted customer experience, cut research and marketing costs, and helped automate business operations.
- Looking For an Integrated Machine Learning Solution?
1. Healthcare
From basic telemedicine chatbots to advanced medical imaging and diagnosis, the healthcare sector has witnessed several revolutionizing applications of machine learning.
ML is powering robotic surgeries, reducing the gap between urban and rural healthcare, improving treatment protocols, and boosting research and development efforts in drug identification and therapeutics.
According to a G2 report, Google’s machine learning algorithm is up to 95% accurate while predicting a patient’s death. The same report suggests that Google’s Deep Learning platforms can detect breast cancers with 89% accuracy.
A popular mental health and meditation app ‘Calm’ is leveraging machine learning to personalize the content for its huge user base. When it first used Amazon Personalize Model in the backend, it noticed a 3.4% increase in app users’ daily meditation activities.
Embrace 2 is an exemplary application of machine learning which detects patterns of stress in the body and notifies the registered caregivers and emergency contacts before an epilepsy patient would have a seizure.
On surface, it is a smartwatch that uses sensors and records the biological changes happening in the body. From a technical standpoint, the smartwatch architecture is based on machine learning and during development, has been fed with large sets of biomedical data – specifically from epilepsy patients to replicate models.
While wearable technology is one specific avenue where machine learning has made a difference, it is also helping with surgeries and radiotherapy planning.
Pharmaceutical giants like Pfizer and Novartis are using Novel Prediction Model and image recognition features to diagnose heart attacks with 87% accuracy and shrink the drug discovery timelines.
For app owners, startups and technopreneurs, ML has opened up countless opportunities to capitalize in the fitness and healthcare app development industry.
The market still has a gap for condition-specific healthcare applications, like Aysa, that would identify symptoms, diagnose diseases, prescribe over-the-counter medicines, and/or set up telemedicine appointments based on the urgency and sensitivity of the case.
2. Automotive
Automotive and transportation are the biggest, most successful use cases of machine learning applications. As much as it has helped with supply chain and quality assurance, machine learning is also empowering the manufacturing side of vehicles.
ML strengthens the efficacy of automobile assemblage by assessing and predicting patterns of risk. Its major functions, speech and image recognition are used for optimizing passenger experience.
As a secondary use case in the automotive sector, machine learning helps optimize road routes. Automotive app development with machine learning applications is further disrupting domains like waste management and on-road traffic management.
Tesla’s self-driving cars and research units are leveraging machine learning models in bulk. Furthermore, the company is deploying machine learning compute, Dojo Systems, for data centers that will scale the performance of vehicles and robots.
Similarly, Uber is making data-driven architectures for decisions for internal and external stakeholders. One of its machine learning models is called Michelangelo – it helps internal teams deploy other machine learning models for financial planning and business management.
Hyundai has developed and deployed SCC (Smart Cruise Control), a machine learning architecture to support drivers and improve autonomous driving.
3. Ecommerce And Retail
Most ecommerce and retail businesses have long started their omnichannel journeys. But technology is one of the fastest growing and evolving business factors now and these shopping businesses need to stay on their toes and adopt every newly-emerging digital touchpoint to maintain the competitive advantage.
Machine learning is one such digital touchpoint and gateway to ecommerce and retail success. Take examples of Walmart, Target, Alibaba, Amazon and Etsy.
Most of these companies have mobile applications with human-like chatbots for customer attendance and engagement.
Alibaba, one of the biggest ecommerce marketplaces from China, has heavily invested in seven machine learning research labs with data intelligence, natural language processing and image recognition processes topping the charts.
Etsy, another ecommerce giant specializing in handmade items, personalized gifts and digital designs, has created three collaborative teams to leverage machine learning algorithms: Applied Machine Learning Team, Machine Learning Systems Team, and Experimentation Team.
These teams create data models, and training modules to personalize within-session product searches for website and app users. These personalization models are applied through image recognition, ad displays, computer vision and similar product recommendations.
Amazon is applying machine learning models from the simplest to the most complex of the company’s operations. Their retail mobile application allows users to search for products via image recognition features.
On an enterprise level, Amazon is using machine learning models to adopt smart and sustainable packaging, using exemplary ML applications blended with user-centric e-commerce development solutions.
A critical analysis of machine learning’s role in the eCommerce and retail industry suggests that it has already reshaped the marketing and sales infrastructure of this industry.
These companies are running better marketing campaigns, targeting and retargeting the right audience via social media ads, improving leads and conversion rates via data analysis, and optimizing delivery routes.
4. Real Estate
Real estate is one of the always booming markets of the global economy. Different domains of artificial intelligence have transformed the way people search for and buy homes and commercial properties.
With the help of machine learning models, real estate companies and apartment data services are feeding their systems with large property-related datasets containing details like area of the property, crime rate, local facilities, mortgage rates, etc. These applications are helping with risk assessment, property valuation, and lead generation.
Popular real estate apps like Zillow and Trulia have deployed machine learning models in their real estate app development architectures to provide personalized property recommendations and help property hunters find their dream homes and office spaces.
In addition, Zillow used SageMaker to create floor plans – one of the most important decision-making tools in the real estate industry. SageMaker is a cloud-based machine learning deployment model powered by AWS.
On the broker/agent side, machine learning applications like conversational chatbots are bridging the customer engagement gap by addressing home hunters’ queries in real time and booking their home visit slots.
5. Space
When applied to space explorations, Machine Learning can help chart new galaxies, discover new habitats, predict solar radiation events, identify asteroids and may be, find signs of new life.
NASA, as an exemplary space and earth science organization and globally recognized for its contributions in the field displays some of the biggest applications of machine learning in space exploration.
It has partnered with tech giants like IBM and Google and brings together Silicon Valley investors, scientists, doctoral-level students and subject matter experts to facilitate NASA’s goals of exploration.
Recently, NASA has spotted around 1.8 billion trees in Africa’s drylands. The associated team is expanding their datasets and neural networks to further understand how these trees can impact the global carbon climate and carbon footprints.
ML can be particularly used for weather and climate predictions on different planets, tracking changes in short and long-term climatic conditions, changes in the overall environmental and biotic factors etc.
6. Waste Management
Enterprise-grade waste management companies are using machine learning to find ‘treasure in trash’ – an ideal metaphor for this use case. In broader terms, their efforts are directed to improve recycling and reusability of waste and reduce the load on landfills.
IBM Garage is IBM’s flagship machine learning framework used by diverse industries and domains. A company called Hera has recently partnered up with IBM to develop waste identification and segmentation datasets to automate company’s commercial waste management processes in future.
As a sustainable packaging challenge, Amazon is using machine learning models to eliminate 915,000 tons of packaging.
The internal departments of the company: customer packaging experience and Amazon Web services have partnered up to make more sustainable packaging systems. This specific effort is part of Amazon’s commitment to The Climate Pledge.
To further propel the application of this innovative technology in waste management, a company called Recycleye has created WasteNet, the world’s largest waste dataset.
The same company has initiated research called brand-level waste management. It is an emerging application of ML’s image recognition feature in which datasets are trained to identify brand logos.
The initial researches used Pepsi, Coca Cola and Heineken’s logos. The model was fed with 1399 random images from the internet.
The test yielded results that were 93% accurate, provided varying lighting conditions, diverse international backgrounds, and mixed shaped visuals of product conditions.
This application of machine learning in waste management can be leveraged by different companies as a contribution to circular economy, while reducing the after-use environmental impact of personal and domestic care products.
7. Banking And Finance
Machine learning applications in finance and banking sector allow for better credit management and loan approvals. Neural networks and machine learning models can identify and analyze prospective lenders’ ability to return the bank’s money.
These algorithms can calculate and analyze with much higher speed and better accuracy as compared to conventional data analysis models still used in a lot of small to medium-sized banking organizations.
Ultimately, these ML-based digital banking solutions are eventually automating credit and underwriting processes.
A report suggests that machine learning predicted stock fluctuations with upto 62% accuracy.
Better credit information supported by machine learning has high impact on firm financing (World Bank).
Machine learning applications can better assess risk, especially when data relationships are non-linear, and analyze the data for small to medium-sized borrowers.
Still a work in progress considering the sector, some organizations have already started applying machine learning models to detect identity theft and fight frauds. But most consumer-side and producer-side fintech apps do leverage machine learning algorithms to send personalized investment recommendations and insights on expenditure and savings.
PayPal, a leading financial institution and digital payment method provider is reported to strengthen the accuracy of its fraud-detection programs.
Though PayPal’s official website does not show a solid figure on what percentage of accuracy these fraud-detection systems provide, it has flooded information on how it’s leveraging machine learning for preventing frauds for its 350 million consumers and merchants.
8. Entertainment
In the entertainment sector, machine learning applications are helping media companies produce what the audience likes, and recommend what the audience is interested in.
Modern machine learning models now keep a close eye on different artists’ social following and measurable influence factors. Then artists with strong social proof in balance with their art skills are featured in the newest roles and movies.
Netflix and Amazon Prime come up with recommendation sections like ‘Popular on Netflix’, ‘Trending Now’, ‘Top 10 in USA Today’ etc. These media companies do so by processing and analyzing large behavioral datasets of individual media accounts.
One of the Machine Learning-related statistics on G2 suggests that Netflix managed to save approximately 1billion dollars while utilizing machine learning neural networks for personalized media content recommendations.
Source: Netflix
Spotify is another fastest-growing music entertainment company that’s leveraging machine learning for personalizing the listening experience.
It processes users’ past behavior, listening, and search history and then recommends songs, playlists, and podcasts to meet personalized requirements.
This company is leveraging a robust machine learning infrastructure called Paved Road and has provided its associated engineers with the tools and frameworks including TensorFlow, Kubeflow, and Google Cloud Platform.
Soundcloud is another example of ML-led music app development that has leveraged offline mode – and does all to maximize the user’s streaming experience.
Wrapping Up…
Tech and non-tech industries have already witnessed the far-reaching effects of machine learning applications.
However, 56% of respondents still face challenges when deploying machine learning algorithms. This is where Tekrevol can make your business automation and transformation journey hassle-free, fully-managed and impactful.
Whether you plan to deploy ML-based chatbots in your mobile app development process, or want to build a fully-functional ML-based business success architecture, Tekrevol has got an A-Team of engineers that knows the pulse of this technology.
These engineers work alongside industry specialists and business strategists who together make your digital success a reality within the designated timelines.
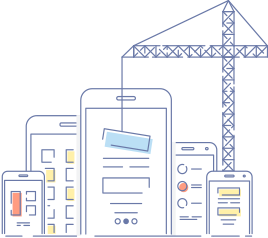
- Partner with Tekrevol to build a Machine Learning App and/or Business Model.